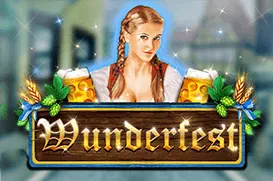
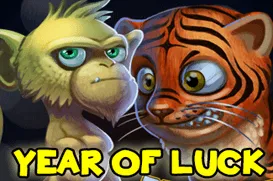
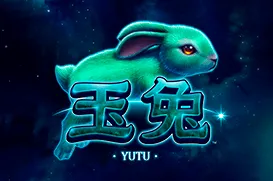
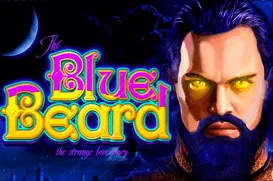
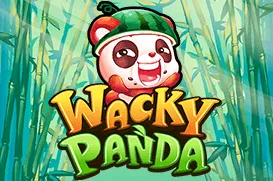
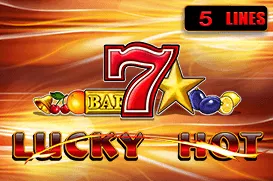
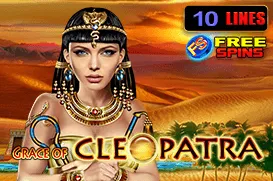
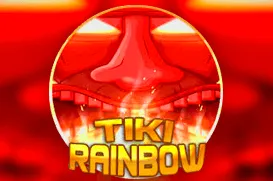
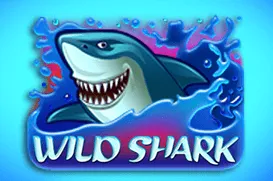
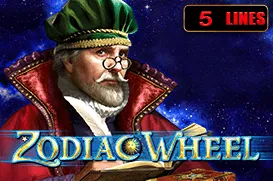
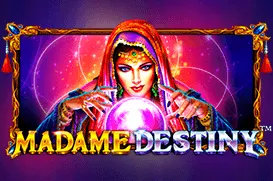
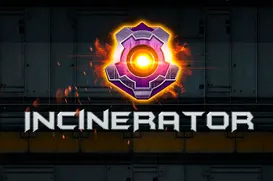
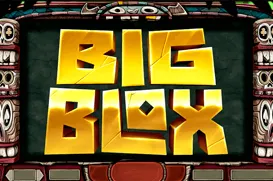
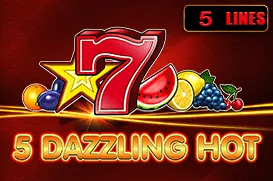
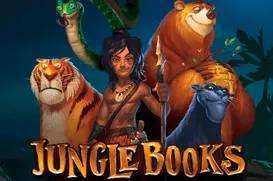
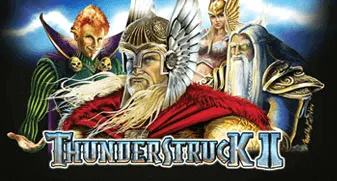
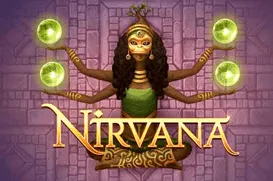
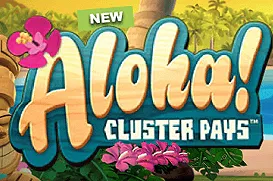
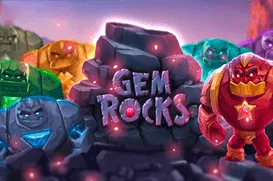
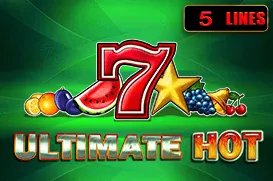
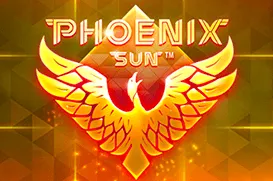
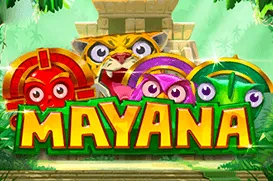
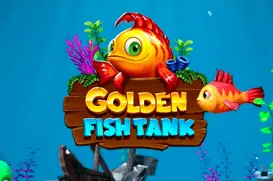
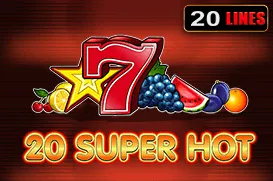
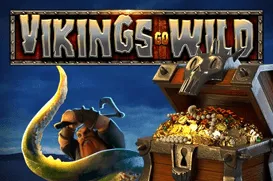
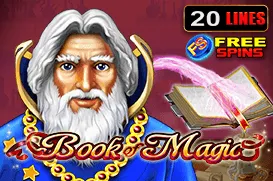
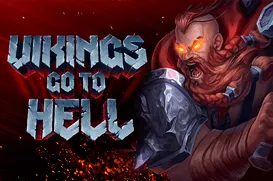
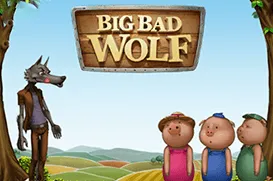
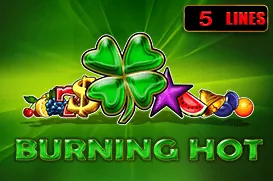
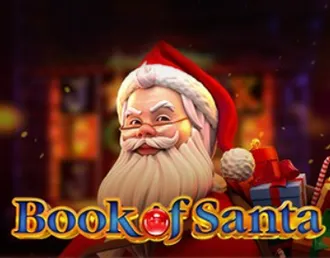
Jet Casino — это уникальная платформа, где каждый игрок найдет развлечение по душе. Здесь представлено множество игровых автоматов, настольных игр и живых казино, которые порадуют как новичков, так и опытных игроков. Разнообразие слотов с захватывающими тематиками и инновационными функциями позволяет испытать удачу и насладиться игровым процессом. Любители классики оценят коллекцию рулеток и покера, а те, кто предпочитает атмосферу настоящего казино, смогут поиграть с живыми дилерами. Jet Casino гарантирует качественный игровой опыт и увлекательные приключения в мире азартных игр.
Джет Казино предлагает игрокам уникальные возможности для развлечения и выигрыша. На платформе представлены разнообразные игровые автоматы, которые удовлетворят даже самых требовательных пользователей. Благодаря высокому качеству графики и звука, каждый слот становится настоящим приключением.
В Джет Казино вы найдете не только слоты, но и широкий выбор настольных игр. Любители классики смогут насладиться игрой в покер, блэкджек и рулетку. Для тех, кто предпочитает живое общение, доступны игры с живыми дилерами, создающие атмосферу настоящего казино.
Jet Casino заботится о безопасности своих пользователей, предлагая надежные методы защиты данных и быстрые выплаты. Игроки могут быть уверены в честности игры и прозрачности всех процессов. Это делает платформу одной из самых надежных в индустрии.
Для удобства пользователей Джет Казино предлагает различные бонусы и акции. Новички могут воспользоваться приветственным бонусом, а постоянные игроки — участвовать в регулярных акциях и турнирах. Это делает игру еще более захватывающей и выгодной.